Everyone building predictive models or performing statistical fitting
knows about overfitting. This arises when the function
represented by the model includes components or aspects that are
overly specific to the particularities of the sample data used for
training the model, and that are not general features of datasets
to which the model might reasonably be applied.
The failure mode associated with overfitting is that the performance
of the model on the data we used to train it is significantly
better than the performance when we apply the model to other data.
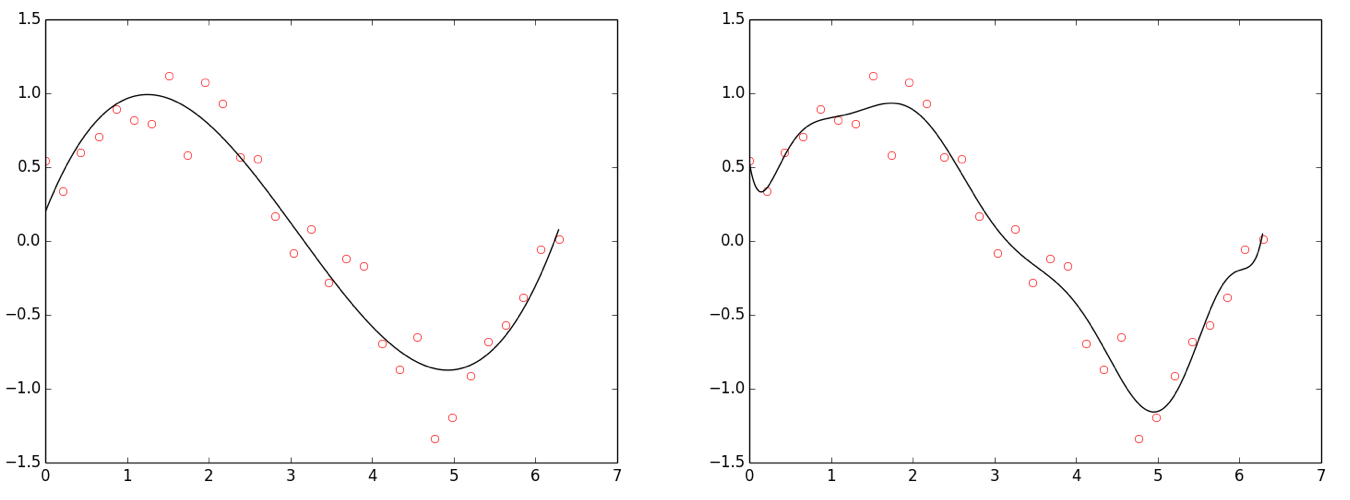
Figure: Overfitting. Points drawn from sin(x) + Gaussian noise. Left: Polynomial fit, degree 3 (cubic; good fit). Right: Polynomial fit, degree 10 (overfit).
Statisticians use the term cross-validation to describe the process
of splitting the training data into two (or more) parts, and using one
part to fit the model, and the other to assess whether or not it
exhibits overfitting. In machine learning, this is more often
referred to as a "test-training" approach.
A special form of this approach is longitudinal validation, in which
we build the model on data from one time period and then check its
performance against data from a later time period, either by
partitioning the data available at build time into older and newer
data, or by using outcomes collected after the model was built for
validation. With longitudinal validation, we seek to verify not only
that we did not overfit the characteristics of a particular data
sample, but also that the patterns we model are stable over time.
Validating against data for which the outcomes were not known when the
model was developed has the additional benefit of eliminating a common
class of errors that arises when secondary information about
validation outcomes "leaks" during the model building process. Some
degree of such leakage—sometimes known as contaminating the validation
data—is quite common.
Generalized Overfitting
As its name suggests, overfitting as normally conceived is a failure
mode specific to model building, arising when we fit the training
data "too well". Here, we are are going to argue that overfitting is
an example of a more general failure mode that can be present in any
analytical process, especially if we use the process with data other
than that used to build it. Our suggested name for this broader class
of failures is errors of applicability.
Here are some of the failure modes we are thinking about:
Changes in Distributions of Inputs (and Outputs)
-
New categories. When we develop the analytical process, we see
only categories A, B and C in some (categorical) input or
output. In operation, we also see category D. At this point our
process may fail completely ("crash"), produce meaningless outputs
or merely produce less good results.
-
Missing categories. The converse can be a problem too: what if a
category disappears? Most prosaically, this might lead to a
divide-by-zero error if we've explicitly used each category frequency
in a denominator. Subtler errors can also creep in.
-
Extended ranges. For numeric and other ordered data, the
equivalent of new categories is values outside the range we saw in
the development data. Even if the analysis code runs without
incident, the process will be being used in a way that may be
quite outside that considered and tested during development, so this
can be dangerous.
-
Distributions. More generally, even if the range of the input
data doesn't change, its distribution may, either slowly or
abruptly. At the very least, this indicates the process is being
used in unfamiliar territory.
-
Nulls. Did nulls appear in any fields where there were none
when we developed the process? Does the process
cater for this appropriately? And are such nulls valid?
-
Higher Dimensional Shifts. Even if the the data ranges and
distribution for individual fields don't change, their higher
dimensional distributions (correlations) can change
significantly. The pair of 2-dimensional distributions below
illustrates this point in an extreme way. The distributions of both
x and y values on the left and right are identical.
But clearly, in 2 dimensions, we see that the space
occupied by the two datasets is actually non-overlapping, and on
the left x and y are negatively correlated, while on the right
they are positively correlated.
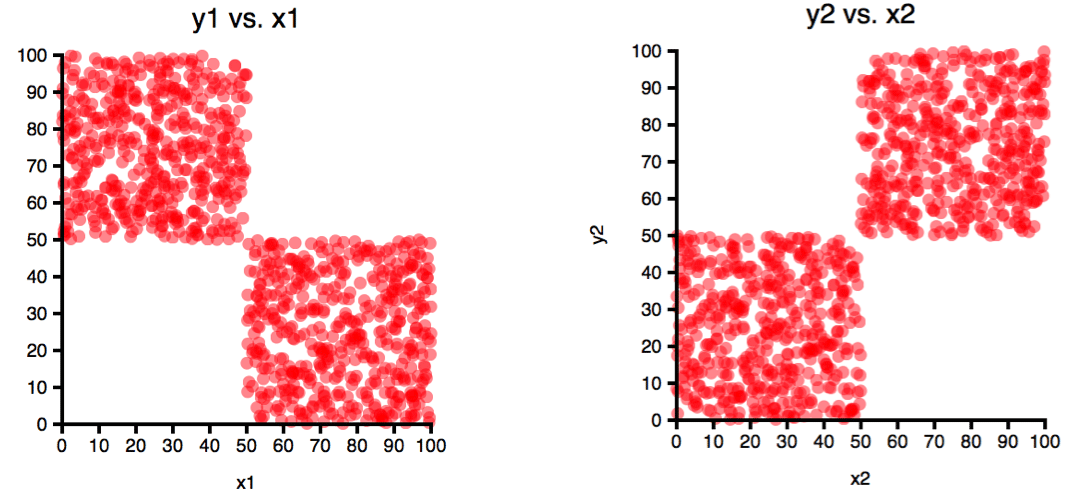
Figure: The same x and y values are shared between these two
plots (i.e. the disibution of x and y is identical in each
case). However, the pairing of x and y coordinates is different. A
model or other analytical process built with with negatively
correlated data like that on the left might not work well for
positively correlated data like that on the right. Even if it does
work well, you may want to detect and report a fundamental change
like this.
-
Time (always marching on). Times and dates are notoriously
problematical. There are many issues around date and time formats,
many specifically around timezones (and the difference between a
local times and times in a fixed time zone, such as GMT or UTC).
For now, let's assume that we have an input that is a well-defined
time, correctly read and analysed in a known timezone—say
UTC. Obviously, new data will tend to have later
times—sometimes non-overlapping later times. Most often, we need
to change these to intervals measured with respect to a moving
date (possibly today, or some variable event date, e.g. days since
contact). But in other cases, absolute times, or times in a cycle
matter. For example, season, time of month or time of day may
matter—the last two, probably in local time rather than UTC.
In handling time, we have to be careful about binnings, about
absolute vs. relative measurement (2015-12-11T11:00:00 vs.
299 hours after the start of the current month), universal
vs. local time, and appropriate bin boundaries that move or
expand with the analytic time window being considered.
Time is not unique in the way that its range and maximum naturally
increase with each new data sample. Most obviously, other
counters (such as customer number) and sum-like aggregates may
have this same monotonically increasing character, meaning that it
should be expected that new, higher (but perhaps not new lower)
values will be present in newer data.
Concrete and Abstract Definitions
There's a general issue with choosing values based on data used during
development. This concerns the difference between what we will term
concrete and abstract values, and what it means to perform "the same"
operation on different datasets.
Suppose we decide to handle outliers differently from the rest of
the data in a dataset, at least for some part of the analysis.
For example, suppose we're looking at flight prices in Sterling
and we see the following distribution.
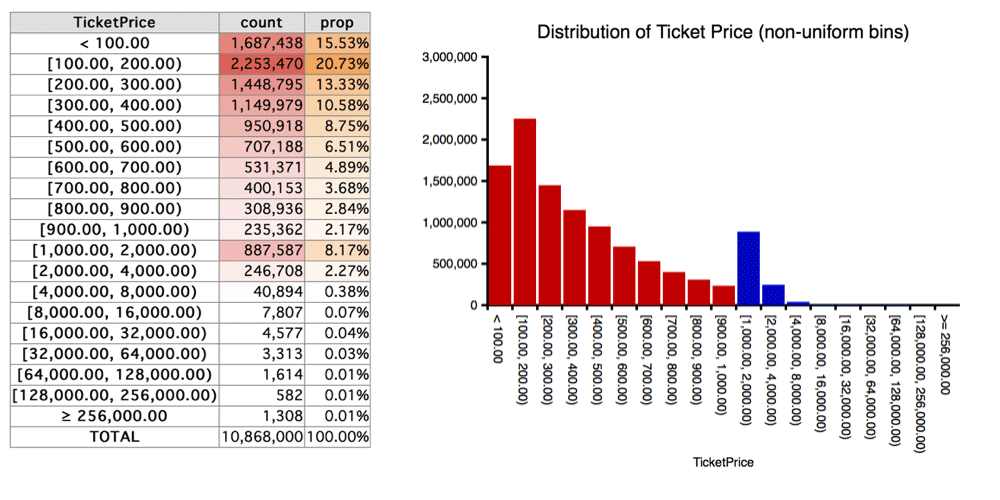
Figure: Ticket prices, in £100 bins to £1,000, then doubling
widths to £256,000, with one final bin for prices above £256,000.
(On the graph, the £100-width bins are red; the rest are blue.)
On the basis of this, we see that well over 99% of the data has prices
under £4,000, and also that while there are a few thousand ticket
prices in the £4,000–£32,000 range (most of which are probably real) the
final few thousand probably contain bad data, perhaps as a result of
currency conversion errors.
We may well want to choose one or more threshold values from the
data—say £4,000 in this case—to specify some aspect of our
analytical process. We might, for example, use this threshold
in the analysis for filtering, outlier reporting, setting a final bin boundary
or setting the range for the axes of a graph.
The crucial question here is: How do we specify and represent our
threshold value?
-
Concrete Value: Our concrete value is £4,000. In the current
dataset there are 60,995 ticket prices (0.55%) above this value
and 10,807,905 (99.45%) below. (There are no prices of exactly
£4,000.) Obviously, if we specify our threshold using this
concrete value—£4,000—it will be the same for any dataset we
use with the process.
-
Abstract Value: Alternatively, we might specify the value
indirectly, as a function of the input data. One such abstract
specification is the price P below which which 99.45% of ticket
prices the dataset lie. If we specify a threshold using this
abstract definition, it will vary according to the content of
the dataset.
-
In passing, 99.45% is not precise: if we select the
bottom 99.45% of this dataset by price we get 10,808,225
records with a maximum price of £4,007.65. The more precise
specification is that 99.447046% of the dataset has prices
under £4,000.
-
Of course, being human, if we were specifying the value in this
way, we would probably round the percentage to 99.5%,
and if we did that we would find that we shifted the threshold
so that the maximum price below it was £4,186.15, and the minimum
price above was £4,186.22.
-
Alternative Abstract Specifications: Of course, if we want to
specify this threshold abstractly, there are countless other ways
we might do it, some fraught with danger.
Two things we should definitely avoid when working with data like
this are means and variances across the whole column, because they
will be rendered largely meaningless by outliers. If we blindly
calculate the mean, μ, and standard deviation, σ, in this dataset,
we get μ=£2,009.85 and σ=£983,956.28. That's because, as we noted
previously, there are a few highly questionable ticket prices in
the data, including a maximum of
£1,390,276,267.42. Within the main body of the
data—the ~99.45% with prices below £4,000.00—the
corresponding values are μ=£462.09 and σ=£504.82. This emphasizes
how dangerous it would be to base a definition on full-field
moments such as mean or variance.
In contrast, the median is much less affected by outliers. In the
full dataset, for example the median ticket price is £303.77,
while the median of those under £4,000.00 is £301.23. So another
reasonably stable abstract definition of a threshold around
£4,000.00 would be something like 13 times the median.
The reason for labouring this point around abstract vs. concrete
definitions is that it arises very commonly and it is not always
obvious which is preferable. Concrete definitions have the advantage
of (numeric) consistency between analyses, but may result in analyses
that are not well suited to a later dataset, because different choices
would have been made if that later data had been considered by the
developer of the process. Conversely, abstract definitions often make
it easier to ensure that analyses are suitable for a broader
range of input datasets, but can make comparability more difficult;
they also tend to make it harder to get "nice" human-centric
scales, bin boundaries and thresholds (because you end up, as we saw
above, with values like £4,186.22, rather than £4,000).
Making a poor choice between abstract and concrete specifications
of any data-derived values can lead to large sections of the data
being omitted (if filtering is used), or made invisible (if used
for axis boundaries), or conversely can lead to non-comparability
between results or miscomputations if values are associated with
bins having different boundaries in different datasets.
NOTE: A common source of the leakage of information from
validation data into training data, as discussed above, is the use
of the full dataset to make decisions about thresholds such as those
discussed here. To get the full benefit of cross-validation, all
modelling decisions need to be made solely on the basis of the
training data; even feeding back performance information from
the validation data begins to contaminate that data.
Data-derived thresholds and other values can occur almost anywhere
in an analytical process, but specific dangers include:
-
Selections (Filters). In designing analytical processes, we may
choose to filter values, perhaps to removing outliers or
nonsensical values. Over time, the distribution may shift, and
these filters may become less appropriate and remove
ever-increasing proportions of the data.
A good example of this we have seen recently involves negative
charges. In early versions of ticket price information, almost
all charges were positive, and those that were negative were
clearly erroneous, so we added a filter to remove all negative
charges from the dataset. Later, we started seeing
data in which there were many more, and less obviously erroneous
negative charges. It turned out that a new data source generated
valid negative charges, but we were misled in our initial analysis
and the process we built was unsuitable for the new context.
-
Binnings (Bandings, Buckets). Binning data is very common, and
it is important to think carefully about when you want bin
boundaries to be concrete (common across datasets) and when they
should be abstract (computed, and therefore different for different
datasets). Quantile binnings (such as deciles), of course, are
intrinsically adaptive, though if those are used you have to
be aware that any given bin in one dataset may have different
boundaries from the "same" bin in another dataset.
-
Statistics. As noted above, some care has to be taken when any
statistic is used in the dataset to determine whether it should be
recorded algorithmically (as an abstract value) in analysis
or numerically (as a concrete value), and particular care should be
taken with statistics that are sensitive to outliers.
Other Challenges to Applicability
In addition to the common sources of errors of applicability we have
outlined above, we will briefly mention a few more.
-
Non-uniqueness. Is a value that was different for each record
in the input data now non-unique?
-
Crazy outliers. Are there (crazy) outliers in fields where there
were none before?
-
Actually wrong. Are there detectable data errors in the operational
data that were not seen during development?
-
New data formats. Have formats changed, leading to misinterpretation
of values?
-
New outcomes. Even more problematical than new input categories or
ranges are new outcome categories or a larger range of output values.
When we see this, we should almost always re-evaluate our analytical
processes.
Four Kinds of Analytical Errors
In the overview of TDDA we published in Predictive Analytic Times
(available
here),
we made an attempt to summarize how the four main classes of errors
arise with the following diagram:
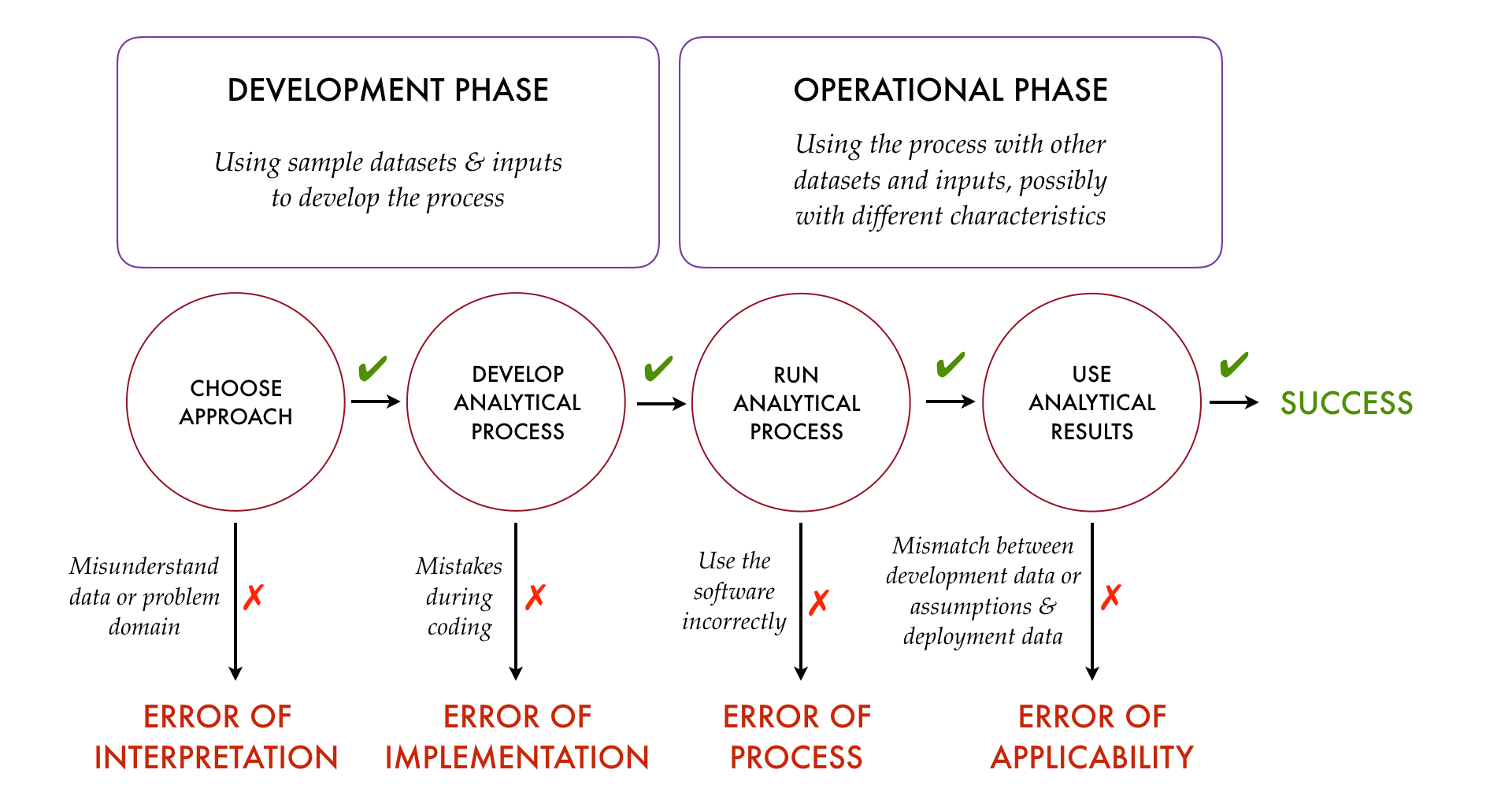
While this was always intended to be a simplification, a particular problem is
that it suggests there's no room for errors of interpretation in the
operationalization phase, which is far from the case.
Probably a better representation is as follows:
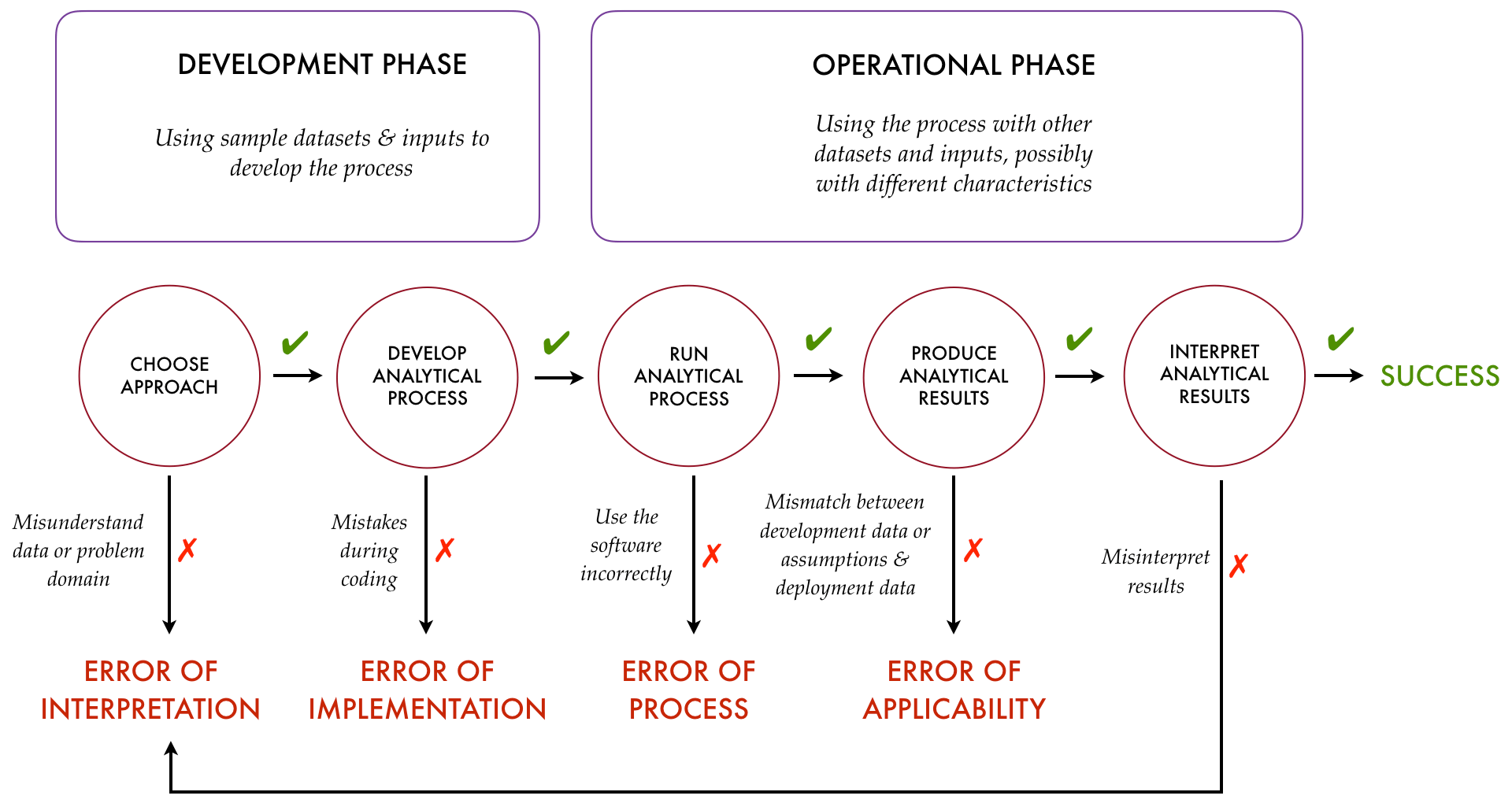